What I’ve Learned from Starting an AI Business
Published on: 12/11/2024
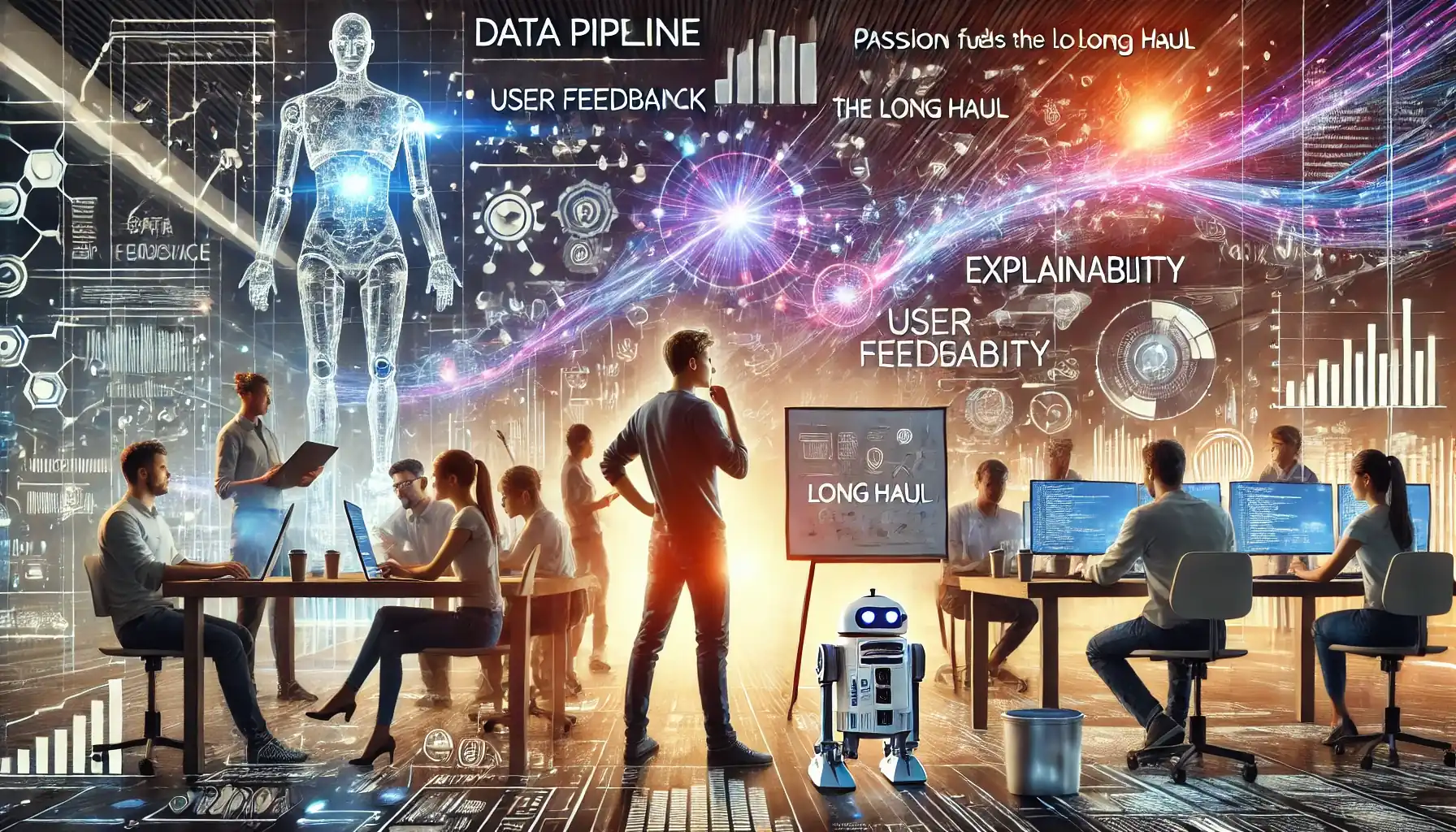
Artificial Intelligence has taken the world by storm, transforming the way businesses operate and how consumers interact with technology. If you’re an entrepreneur—or aspiring one—you’ve probably felt the allure of diving into the AI space. I certainly did. When I first started an AI-focused business during this heady, hype-driven boom, I expected challenges, of course, but not in the exact shape or scale they ultimately revealed themselves. AI, as cutting-edge as it is, comes packaged with unique obstacles, surprising twists, and a learning curve that doesn’t just vanish after launch.
Today, AI is everywhere—language models that draft emails, image generators that create art from a few text prompts, predictive algorithms that foresee customer churn, you name it. The gold rush into AI startups has inspired countless entrepreneurs, and along with them, a wave of investors, analysts, and advisors. While there’s no shortage of thought pieces on how to break into the AI industry, there’s something about learning directly from the trenches. As someone who jumped right into the deep end, I’d like to share what I’ve discovered.
Below is a ranked list of 17 lessons—each encapsulated in a single, punchy one-liner—followed by some commentary drawn from my own experiences. These insights come from my successes, my missteps, and the times I had to pivot, rebrand, or rebuild from scratch. If you’re looking to launch your own AI business or refine the one you have, I hope these lessons serve as a helpful guide, or at least a cautionary tale.
1. “Hype Is Not a Business Model”
It’s easy to get swept up in the whirlwind of excitement and media buzz around AI. Headlines tout AI as the next electricity, the next internet. While the hype can help you score initial interest or funding, it’s not something you can build a sustainable operation around. All that excitement can attract early customers, but what they really need after the initial spark is tangible value. Don’t mistake the industry’s hype for a guaranteed path to product-market fit. Real customers stick around for solutions that make their lives easier, not because the tech has a catchy acronym.
2. “Your Data Pipeline Will Make or Break You”
AI thrives on data—good, clean, well-structured data. Without it, even the best models fall short. I learned the hard way that understanding and meticulously managing your data pipeline is crucial. From collection and cleaning to validation and storage, handling data properly can mean the difference between a working product and a malfunctioning demo. If you skimp on data management, you’ll spend countless hours firefighting, trying to figure out why predictions suddenly went off the rails. Invest early in robust data engineering practices.
3. “Customers Don’t Care How Smart Your Model Is—Only How It Helps Them”
As technologists, we love to brag about model accuracy, algorithmic complexity, and performance metrics. But customers rarely care about the intricacies under the hood. What they want is to see tangible results: improved efficiency, reduced costs, better user experiences. If you obsess over the cutting-edge brilliance of your AI but fail to translate that into user value, you’ll find people tuning out. Always frame your AI’s capabilities in terms of outcomes and impact.
4. “Explainability Isn’t Just a Buzzword—It’s a Trust Builder”
In a field infamous for its black-box solutions, explainability becomes a selling point. Clients want to know why your AI recommends one decision over another. Embrace interpretability tools and make user-friendly dashboards that offer clarity. By pulling back the curtain, you earn trust. Trust fosters loyalty and turns skeptical leads into long-term customers. Without explainability, your product can feel too risky, too opaque, and not worth the investment.
5. “Your Engineers Need to Speak Business, and Your Business Folks Need to Speak AI”
Cross-functional understanding is the secret sauce. Engineers who grasp the commercial implications of their technical choices can prioritize features that drive revenue. Sales and marketing teams who understand AI’s constraints and capabilities can set realistic expectations with clients. If your team can’t communicate across these divides, you’ll end up with misaligned product features, overpromised capabilities, and frustrated customers. Bridge these gaps through regular workshops, documentation, and a culture of curiosity.
6. “Talent Wars Are Real, and They’re Brutal”
The demand for top AI talent is relentless. When I started, I naively assumed I could just hire a few data scientists and be done. Instead, I found myself competing with tech giants and well-funded startups for the same small pool of experts. To attract and retain great people, you need more than a competitive salary. Offer interesting problems, professional growth paths, and a positive culture. The talent you secure will become the backbone of your business.
7. “User Feedback Is Your Best Tuning Parameter”
No matter how much offline training or simulation you do, nothing compares to the insights you glean from real user feedback. Users will spot gaps and uncover use cases you never thought of. Continuous iteration based on their input allows you to refine your models and sharpen your product-market fit. Don’t just collect feedback; actively seek it out. Make it easy for users to share their thoughts. Iterate, deploy, listen, repeat.
8. “Infrastructure Costs Can Be a Silent Killer”
AI workloads aren’t cheap. Training large models and running inference at scale can lead to infrastructure bills that balloon over time. Early on, budget for these costs and track them religiously. Use cloud credits, optimize model architectures, and consider when to buy vs. build. Don’t let a promising margin vanish under the weight of GPU bills and scaling challenges. Keep an eye on cost-effective frameworks and tools, and never assume that throwing more computational power at the problem is always the best solution.
9. “Partnerships Can Accelerate Everything—Or Drag You Down”
Teaming up with other companies can give you a head start: access to data, distribution channels, or complementary technologies. But choose your partners wisely. A misaligned partnership can drain resources and introduce complexity. Before committing, be crystal clear about roles, responsibilities, and mutual goals. You want synergies, not compromises that slow you down. The right partner is like a growth catalyst; the wrong one can be a lingering, expensive distraction.
10. “You’ll Need More Than One Monetization Strategy”
Relying on a single revenue stream makes you vulnerable. Maybe you start with a per-seat subscription model, but consider expanding into usage-based pricing, enterprise licensing, or even a marketplace. Diversification is key in a fast-moving industry. Trends shift, and what’s profitable today might be commoditized tomorrow. Stay nimble and ready to adapt your pricing. Experimentation can reveal surprising revenue streams that stabilize your cash flow and secure your future.
11. “Think Global, But Don’t Ignore Local Nuances”
AI is borderless, but markets aren’t. Cultural differences, language barriers, and regulatory frameworks vary wildly by region. Don’t assume that a product that thrives in one geography will automatically scale elsewhere. Understand local data protection laws, partner with regional experts, and consider how cultural factors influence user behavior. By tailoring your approach, you can make your product truly universal without stumbling over local misunderstandings.
12. “Regulation Isn’t a Roadblock—It’s a Road Sign”
The world of AI is becoming more regulated, and that’s not a bad thing. Regulations guide ethical standards, data privacy, and accountability. Instead of viewing compliance as a headache, treat it as an asset. Meeting (and surpassing) regulatory requirements can be a differentiator, reassuring customers and investors that you’re a responsible player in a market still mired in uncertainty. Understanding the rules of the road lets you steer confidently instead of swerving at the last minute.
13. “MVP Means Minimal Viable Proof—Not Just Product”
In a technical sense, an MVP (Minimum Viable Product) for an AI startup might be a stripped-down model that proves the concept works. But it’s not just about the product—it’s about validating the business case. Does anyone actually need what you’re building? Will they pay for it? Your MVP should answer these questions quickly. If you can’t prove the concept’s value to actual users, no amount of feature polish will save you. Speed to validation matters more than perfection at the outset.
14. “Your Brand Is the Story People Tell When You’re Not in the Room”
With so many AI startups flooding the market, how do you stand out? Your brand. It’s the promise you make to customers and the narrative you tell about your mission, values, and capabilities. A strong brand creates emotional resonance and trust. Invest time in creating a consistent voice, visual identity, and messaging that differentiates you. When customers and investors think of your company, you want them to recall a story of innovation, reliability, and human-centric design—not just a generic AI widget.
15. “Scaling Is About Processes, Not Just Models”
It’s one thing to build a great model and land a few clients. Scaling means being able to onboard new customers rapidly, integrate feedback loops, maintain version control, and ensure uptime. Processes are your secret weapon. From QA protocols to deployment pipelines, the more standardized your operations, the easier it is to grow. Remember that scaling isn’t just a technical challenge; it’s also an organizational one. Solid processes free you to focus on innovation rather than constantly putting out fires.
16. “Adapt Fast—The AI Market Doesn’t Wait”
The AI landscape shifts rapidly as new breakthroughs emerge and yesterday’s breakthroughs become today’s table stakes. If your product isn’t evolving, it’s stagnating. Be ready to pivot, rewrite code, and revisit your go-to-market strategy. Agile methodologies help, but so does fostering a company culture that embraces change rather than fears it. Keep a close eye on research, competitor moves, and emerging technologies. Adaptation and continuous learning are the only surefire ways to avoid obsolescence.
17. “Passion Fuels the Long Haul”
AI is a marathon, not a sprint. While passion alone isn’t enough, it’s the fuel that keeps you running through late nights, tough deadlines, and unexpected setbacks. Without a real passion for your problem space and the technology, you’ll find it hard to stay motivated when the going gets tough. Passion helps you push through the hype cycles, the talent wars, and the regulatory hurdles. It keeps you curious, open-minded, and driven. And in a field as dynamic and challenging as AI, that might be your most valuable asset.
Conclusion
Starting an AI business during the boom is a thrill ride. The potential upside is massive—imagine being at the forefront of an industry that could reshape the world. But the journey is fraught with obstacles and hard-earned lessons. Hype isn’t a substitute for strategy; complexity demands transparency; and customers only care if your product actually solves their pain points. Mastering the fundamentals—data pipelines, trust-building, effective talent management—and remaining flexible in a hyper-evolving landscape can set you apart.
Above all, remember that at the end of the day, AI is a tool. It exists to serve human needs and solve human problems. Keep that humanity front and center as you build. If you do, you’ll not only create a product that resonates with users, you’ll also ensure that the time, energy, and resources you invest in this space pay off in the long run.
Starting an AI business during the boom is a thrill ride. If you’re interested in seeing how AI can transform productivity, check out our Sharpen Chrome extension, Sharpen Notes to learn more.